Leveraging
AI and CX
for
High-Impact
CDP Use Cases
Achieving breakthrough outcomes, especially in just 83 days, is only possible through seamless collaboration. At our MarTech and Data Solutions Center of Excellence, we combined the strategic insights of a Senior CX/UX Strategist and the analytical prowess of a Data Scientist to create a game-changing solution.
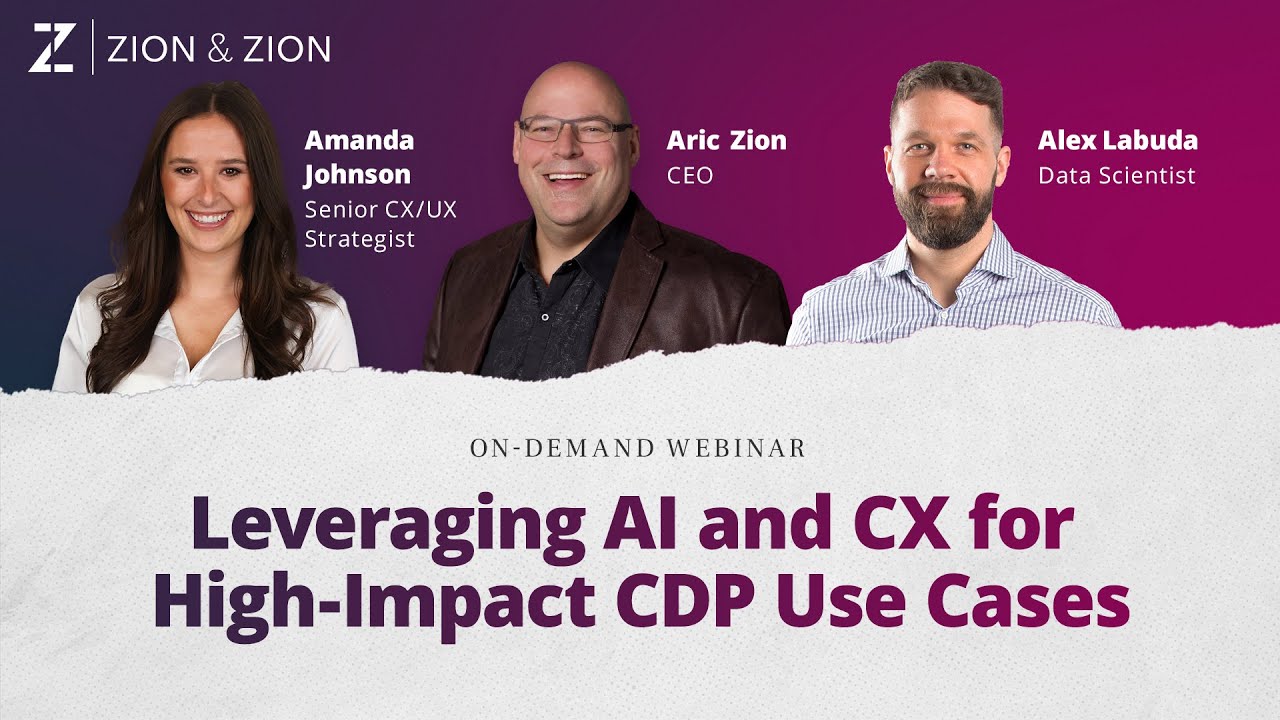
Key Insights
- Our team brought together CX and data science expertise for rapid innovation.
- The project was executed in just 83 days—a remarkable timeline for this level of complexity.
- This kind of cross-functional collaboration ensures success in even the most challenging and data-driven projects.
Aric:
Well, thanks everyone for joining us today. I think we have a really exciting webinar, a very exciting project the agency has worked on and is still working on. And today we have with us to discuss that, two members of our MarTech and Data Strategy Center of Excellence, which is a heavy customer data platform focus within that group.
One is Amanda Johnson, who is a Senior CX/UX Strategist in that Center of Excellence. And the other is Alex Labuda, who is part of the Data Science and AI Engineering Group within the MarTech and Data Strategy Center of Excellence. So good morning to both of you.
So let me flash up for a second, a chart that we are then probably barely going to reference as we go through the webinar, because the chart is the architecture of the system that this team plus a bunch of other people on our team has built, but the focus of today’s webinar, and you can see a fairly complex architecture on the screen now, but the focus of the webinar is actually not the architecture. The focus is the degree of collaboration and interaction it took across this Center of Excellence within the agency in order to achieve this objective, right?
I mean, architectures can be built all day long, actually execute them and building them around use cases. We feel is so often the people in process part that is so hard compared to the technology part. And that’s really what today’s webinar is gonna focus on is how Amanda and Alex, as well as many others on the team collaborated over an 83 days, right?
Do I have that number right, guys? Right, 83 days to build this prototype, which for us and probably for most people out there is some sort of a record. So Amanda, why don’t you tell us about what this whole project was about?
Amanda:
What were we trying to do for this client in the CDP plus AI, et cetera world? Yeah, so the client was Closet Factory and really how this started was we discovered that there was so much good zero party data coming from customers within their call center. So their customers are calling in, they’re talking to a customer service rep about their project.
And there’s so much valuable information in these sometimes 30 second calls, sometimes five minute calls that there’s just no possible way a CSR could manually get all of the rich data that’s coming directly from these customers. So really we were looking for a CDP strategy to build out CDP use cases built upon the kinds of zero party data we noticed we were getting from these calls. I think it’s really valuable to kind of hear firsthand the kind of data that we were hearing from customers.
So we actually have a short clip that we’ll play. That’s just a quick recording of one of our calls. We’ll just play a little bit of that so you can kind of get an insight into the types of information we would hear from a customer.
So we’ll go ahead and play that now.
[Audio Recording Plays]
CSR:
Thank you for calling Closet Factory. This is Sue. How may I help you?
Customer:
Hi, I’m looking to get my closet done.
CSR:
Okay, yeah, we can definitely help with that. Is it a walk-in, is it a reach-in closet?
Customer:
Yes, we have a walk-in closet, but I’ve got a few questions first.
CSR:
Okay, yeah, absolutely. I can help with that.
Customer:
Okay, so what does the process look like? I guess I’m wondering if someone’s gonna come to my house or is there a showroom somewhere nearby that I should plan to visit?
CSR:
Yeah, so you’ll be paired with a professional designer who will help you design your space. They’ll come to your house, they’ll take measurements, inventory all the items in your closet, all before creating a design for you.
Customer:
Okay, okay, cool. So you’re gonna bring examples of wood colors and other materials when you come to? I’ve been thinking about a specific design, but I really wanna make sure it fits my vision.
CSR:
Yeah, we’ll bring samples. We have the largest selection actually of styles and colors and finishes in the industry. So you’ll have lots to choose from.
Customer:
Okay, great, I love choice and I do want a professional designer to give recommendations to fit everything in. We’re in a condo, so there’s not a lot of space and really I need someone to help us organize it.
CSR:
Our designers are great at doing that. They’ll talk with you about what you’re wanting, give you some ideas of how to maximize your space. So we can absolutely help with that.
Customer:
Okay, okay, great. Do you have any deals that you’re running right now?
CSR:
Oh, like discounts, yeah. We actually happen to have a 15% off offer running right now for new customers.
Customer:
Great, okay, I’m still thinking about it and if it’s something I can afford right now, it might be something I have to wait on, but a discount obviously would really help.
CSR:
Totally understand that. Our closets, you should know, are fully custom. So when you work with your designer, you can create something that’s within your budget, whatever you’re looking to spend.
Customer:
Oh, good to know. Okay, I think I need to think about it a little more and I’ll probably call back when I’ve talked to my husband and we’re ready to make an appointment.
CSR:
That sounds great. Thanks for calling. We look forward to hearing from you.
[Audio Recording Ends]
Aric:
All right, thank you. So, wow. Yeah, I mean, there’s definitely a lot of information contained in that conversation there.
So Amanda, why don’t you talk to us about what kind of information, what buckets, what structure? I’m gonna let you go through that, that’d be great. Yeah, so a few of the things that we heard on that call that we can kind of deduct that we would consider zero party data that would help drive some of those use cases that we would develop as a result of this.
Amanda:
So we’ll look at this here, we have project information. So the customer told us about their space, that it’s a condo, so it’s a smaller space. They also told us at the end of this call that they’re not quite ready to book yet, that they’re still in that phase of gathering information.
They’ve also told us that they are design focused, that they care about the design and the different colors and wood samples and that they can really customize their space with the different materials. They’ve also mentioned that they care about the process. They asked, what does this process look like?
So that tells us that they care about the order of operations and that we need to give them a little bit more information and insight into that. And then they also told us that they were price sensitive. They asked questions about deals and discounts.
And so that tells us that more of that price sensitive information or that discount messaging would really resonate with this type of audience more. So all of that gathered in this short call, again, there’s really no way that a CSR, they could gather some of this information, but there’s just really no way they could capture all of that. So really after listening to these calls and seeing all this information, that’s really when it became apparent that there was a need for, or an opportunity, I should say, for AI to really come in and help drive the CDP strategy.
Aric:
Got it. And am I correct, as I recall from this project, sure, there was all this zero party data in these calls, but your work with the client and the client’s team started much earlier than let’s dig out an AI engineer around the zero party data in the calls, right? Absolutely, yeah.
So I would say what kind of drove us to knowing even what this information means was months before of doing work on the customer journey side on the CX side, we went through a full customer journey mapping process to look at what the touch points throughout the journey from the pre-purchase stage, purchase, post-purchase stage that customers are interacting with, what kind of touch points we could add into that journey that didn’t currently exist, what the thoughts and feelings were of the customers that are going through each of those steps.
So really that was the groundwork that we built upon that as we started to figure out, okay, this call center, this call that a customer is making into Closet Factory is such a key part of the journey. So many thoughts, so many emotions happening at this phase. That’s really what drove forward the strategy to kind of get this project off the ground.
Got it. So then when you have the journey mapped and we’re figuring out what information was relevant, et cetera, I know that two of you, as well as other people in our Center of Excellence had a lot of work, right? You had to dig out info from the calls and do a lot of hand labeling in order to then train the models.
I mean, Alex, maybe you wanna talk about that a little bit, what that process was like, some of the challenges there.
Alex:
Yeah, yeah, absolutely. So I really started from more of a bottom-down approach, and just to kind of learn more about what information we could actually extract from these calls. So this involved like your more traditional text analysis and exploratory data analysis.
And then coming together with the work that Amanda had already completed on the customer journey, we were able to ideate on classifications that would be more valuable to the client and relevant to this particular client’s use case.
Aric:
Got it. So am I hearing correctly that from the journey perspective, you guys worked top-down from the what is possible from the AI engineering, fine-tuning the models, etcetera’s perspective, you worked from bottoms up and then found that sweet spot in the middle?
Alex:
Yeah, yeah. So I will say, I think it’s sometimes what you think you could extract from these calls isn’t always true. You might wanna know a person’s age group or home size, but after doing some exploratory analysis on the data, you might find that this information just doesn’t exist and it’s not possible to extract.
But you can still extract a lot of contextually rich information. And I think understanding the customer’s pain points and their touch points, you can really start to evolve what type of information you’re extracting to make sure you’re creating the most value for the client. So this could be things like their purchase intent or what buying phase they’re in, or even the timeliness, whether they’re ready to purchase now or six months from now.
So this can all influence creative and the campaigns that we use for these customer segments.
Aric:
And I think it’s really interesting because certainly there are called tracking systems out there that off the shelf can listen for keywords and things like that. But what I’m hearing you say, and what I think is really key to this use case is that you were looking for information that was buried in context, those calls. And that like, would it be fair to say something like whether somebody is concerned about budget can’t just be detected by whether they mentioned the word budget or cost.
They might say, my budget’s unlimited. I’m not worried about costs or they might be expressing concern about it. So you have to think about those subtleties.
So that’s really contextual information, which came out of your fine tuning of the LLMs, right?
Alex:
Yeah, exactly. And it also allows us to really customize what segments we want or the various levels of that classification, right? So it might be really important for you to define a particular transcript or a customer, their price sensitivity to be like, high, medium or low, not just true or false, right?
So depending on how granular you need to get for your particular use case, we can really customize that. Whereas your more traditional call tracking might not be able to do so.
Aric:
So question for you. So it wasn’t just the two of you listening to a few hundred calls. There were other people in the Center of Excellence doing this on our team.
How did you guys align on common definitions in these ambiguous circumstances of what something meant so that you all would arrive at the similar data set graded on the same kind of scale?
Amanda:
Right, yeah. So just to start, it was not just Alex and I that worked through this project. We had solutions architects, data engineers, a creative team, data scientists, CX team.
We had across the board. So just like you said, kind of aligning on what does high price sensitivity mean? What are each of that?
So I would say that actually took a few rounds of actually reading through the transcripts and kind of coming up with our own definition to say, if they mentioned this specifically, that I would consider high. So coming up with a few examples and then kind of talking through that, I think there was a ton of collaboration that happened and hearing, especially because there were so many different teams involved, I think just getting the different perspectives from those different angles made this project so much more valuable than if I were working in a silo or if Alex were working by himself, to talk through that with different perspectives and different types of team members, I think really helped us get us to a lot more mature state at the end of this project than if we were working independently on something like that.
Alex:
I really can’t emphasize enough the importance of having a really high quality, accurate data set. So, and making sure that every team member can align and really understand the definitions that of these different classifications.
And in that you might end up having to revise what your classification levels are. You might initially go into this project thinking that you can classify price sensitivity at four different levels, but then find when you’re actually labeling the data set that it’s much harder to define. And particularly with LLMs, you have to make sure that those definitions are very clearly defined.
So I would say, start from more of generalized classifications. Maybe you start with price sensitivity, only having two levels and see how you can perform there. And then given the fact that Amanda and I had the opportunity to work really closely together, we could decide what the value of getting a particular classification wrong was.
Like how much would this affect the client if our LLM made an incorrect guess at what this call transcript should be classified as. And that can really, making sure that you assign business value to these objectives, really helps prioritize the different classifications and how granular you need to get.
Aric:
Got it. So, I mean, really, really interesting. And my, how did you wind up deciding?
Cause obviously, well, maybe not obviously, you didn’t just use an API and pump this over to GPT and send it a prompt and say, figure this out for me. Because I think early on, right, you guys knew that is not going to get you quality. You had to fine tune the LLMs through multiple rounds.
Alex, why don’t you talk to us about that? And I think you brought a chart as well to kind of visualize that.
Alex:
Yeah, absolutely. So, once you kind of derived an accurate training dataset and you have labeled all these different classifications that you want, price intent, what buying phase this particular person might be in, whether it’s new or existing business. Once you get there, it’s usually best practice in any machine learning project to establish some baseline performance, right?
So, what this means is essentially just making all of your classifications, maybe testing different models. So, in this case, testing various LLMs. So, we use some Palm 2, Google LLMs, Gemini, some OpenAI GPT Instruct models. And you establish a baseline of performance for each of those models.
At that point, you basically will iterate on your prompt. So, prompt is essentially some instructions that you give an LLM along with the transcript, and then you ask it to make the classification on the transcript. And the Palm 2 Text Unicorn model ended up outperforming all the other models.
So, that was the model that we ended up going with. In addition to that, most of the infrastructure for this entire project was on GCP, Google Cloud Platform. So, it allowed us for a more seamless integration to use this model.
And once you establish your baseline, then you can decide, okay, we no longer want to approach the path of using Gemini or these other models. And then you kind of work on your prompt engineering. So, I’m going to pull up a slide here to kind of show you what this modeling iteration process sort of looks like.
And here we go, here’s the slide. So, once you’ve established your baseline performance, typically what you’ll do is you’ll look for ways to improve this. And a really good approach to do so is to examine where your model was wrong.
So, you look at the classifications that the model got incorrect, and then you start reading the transcripts and you try to figure out if there’s a pattern. Usually when you look at say four or five different errors, you’ll start to figure out that there is a pattern in the errors. And then you work on refining your prompt to kind of clarify what these errors could have been.
And again, once you’re reviewing them, it becomes kind of obvious. And then you iterate on this process several times. And then depending on the performance, whatever your thresholds were going into the project, let’s say for example, the success criteria was accuracy of 90%.
You’ll decide if this particular classification such as purchase intent or whatever buying stage the customer is in, you decide whether you need to engineer further and iterate more or explore other approaches, which this could be things like few shot prompting. And few shot prompting is essentially the process of giving the LLM some examples. So this is a transcript where the price intent or the purchase intent was X.
And this is an example where the purchase intent was Y. And this really helps the model kind of learn specifically what you’re looking for. And then from there, you might also explore fine tuning.
Let’s say for example, if a certain classification didn’t meet that criteria of 90%. And fine tuning is really the process of giving an LLM and almost retraining it on your data specifically. So you may be looking for a very nuanced classification such as design interest or process analytical, like we wanna understand, does this customer seem to be more interested in the process or are they more interested in the design?
So this could be pretty nuanced for a model to understand with simply few shot prompting or just prompt engineering alone. So, and depending on the business value that this would add, we would then make the decision whether or not we should explore the process of fine tuning our model to the specific data set that we have.
Aric:
Got it. Well, this is really exciting work. Okay, so now kind of unveiling here, all of this work was done to understand the data, analyze it with AI that we did prompting, fine tuning, et cetera, build that architecture, right?
Other members on the team built that architecture all from scratch. But ultimately the goal was customer data platform use cases. So Amanda, I guess back over to you to talk about some of those use cases and then kind of what that looked like.
Amanda:
Yeah, so yeah, definitely a CDP strategy was the output of all of this. So tons of work and gathering that data and some really cool things that we can learn. But ultimately what we derived from this was four use cases that we’ll look at today.
That kind of gave us different perspectives of based on what we’re hearing on a call, how we would bring that into a CDP and classify those different elements. And then what the activations would be as a result of that based on these different audience groups. So I’ll pull those up and we can look through the four of them.
And also we’ll have some examples of creatively how that comes out from the customer’s point of view and what kind of messaging and creative assets came as an output of what these use cases are. So this first use case we’ll look at here, what we’re looking at is a new customer who did not book an appointment. And over on the left hand side, you can see we have the call transcript.
So some of the things we would have heard from this customer when they called in would be things like, I’m redoing my master bedroom closet in my condo, mentioning something like you’re more expensive, asking a question about the process and then ultimately at the end of this call, not booking an appointment. So the classifications that result from that would be this is new business. It’s a closet.
They told us it’s a condo. So we’re able to imply from that that it’s maybe a bit of a smaller space. They’re information seeking because they did not book an appointment at the end of this call.
They’ve told us they have some price sensitivity and they also have mentioned the process. So we’re going to also apply that they have that more process analytical focus as well. As a result of that, we can see four activation channels of how this would play out.
So on the media side, front display, Facebook, Instagram, prospecting and retargeting campaigns with messaging specific to maybe those discounts or values for the costs. We could also personalize their web experience. So as they visit the website for the first time, they’re getting that consistent messaging between those media campaigns and what they’re seeing on the website.
Of course, email, nurture streams and then SMS as well. So then the creative output of this, we can see is this an example of a media campaign. So if they were on Facebook, they’ve told us they are more of that price sensitive.
So we’re going to leave more with that discount messaging so we can see that coming out. And then transitioning to the web experience, carrying across that same discount messaging so that you’re creating a really cohesive experience for that customer across the different touch points. So that’s the first use case.
We’ll go through just a couple more quickly. The second use case here, we can see a slightly different type of customer where this customer is calling in and they’re mentioning that they want to redo their garage. And they’ve also asked questions like, what kind of add-ons do you guys have or what kind of customizations?
And then as a result, they do actually end up booking an appointment. So classifications you can see have slightly adjusted for this customer. They’ve told us it’s a garage, so we can adjust that imagery to be focused to the project they’re interested in.
They’ve told us it’s a house. They’ve also actually taken an action as a result of this call. And then they’re more design focused.
We know that because they mentioned those customizations. I think one important nuance to point out here with because this customer actually took the action to book the appointment, we don’t need to push that messaging as much about discounts or trying to get them to book an appointment because they’ve already taken that. So as we look at the activations and the creative to come out of that, this first example we’ll see here again, media example on Facebook, we can see that the messaging has really shifted more to the largest selection of styles, finishes and accessories.
So we’re really speaking more to that affirmation of their decision to move forward in the process that they’ve already done versus trying to get them to book that appointment. And then another example here on a different platform, something like house, bringing in that garage imagery, bringing in messaging again, just about that design. We’ll look at one more just really quick here.
This third use case, this is an example of an existing customer who is maybe coming back for, they just built a new home and they’re wanting to get all of the closets done in their new house plus their kitchen and their hall. Closet as well. And then they’re also asking questions like, how long can I expect before this work to get done?
So this is an existing business customer. It’s a new home, which is a new build. That was one of the interesting kind of classifications that we through reading transcripts learned that we would be able to deduct, which would come out in the messaging and the imagery.
There was a lot of cool opportunities with that. And then also a unique classification we haven’t seen yet is time urgency. So that really tells us that in the messaging, we can speak more to that time aspect of, hey, we can get this done within the timeframe that you need us to.
And really speaking to that aspect, if we know that’s important for our customers. And then with this, just one thing to point out here on the activation side is you’ll see under that media, really a key thing here was suppression. So because we know that this is an existing customer, but they have new interest, we’re gonna suppress them from any previous messaging about first time customers or anything that wouldn’t resonate with that customer base, because we know that they’ve already worked with us in the past.
So that’s something we can learn from these calls as well. Looking at the creative examples for this, you can see that we’re, because they’ve told us they’re looking back at, or they’re looking into doing more like pantries or things in their kitchen. You can see that coming out in the imagery, the messaging being welcome back.
We know they’re an existing customer. And then of course on the website, bringing in that personalized web experience to bringing in that same messaging and imagery across there as well. So that’s just three examples of the many use cases we could deduct.
I think that what we started with was, we saw a bunch of transcripts. And so we tried to build out these use cases for ones that were the most prevalent or really captured a large amount of their audience base.
Aric:
This is really amazing. I mean, when you think about it, a lot of orgs, their usage of customer data platforms and their audience construction is very limited to, well, what products did you buy before? What products did you look at? Are you a high spending consumer or low spending consumer, a frequent one or not frequent one?
And it can definitely be hard to figure out tailored journeys just based upon that kind of information you guys have gone and the team, because I want to say there were eight or nine people that worked on this and built the prototype. And again, 83 days. So around the world, around this world in 83 days.
And the contextually rich information, the subtlety of what is on the clients, the customer’s mind is so unique to this use case as opposed to just inference from, I looked at A or B or C, gee, what do I do with that, et cetera. So congratulations to you. I know that the client is excited about the progress as well.
And to all of those in the center of excellence, Martech Data Strategy Center of Excellence, we have that are not on this call that worked so hard on this. Thanks to them as well. And I guess we can wrap it up there.
And thanks again for your time, your hard work and all your innovation and collaboration. Thank you.
Alex:
It was very exciting. Thank you, Aric. Thanks everyone.